Artificial intelligence for batteries
A battery requires several manufacturing steps: slurry preparation, coating and drying, electrode calendering, electrolyte deposition, and solid electrolyte interphase formation. This process requires an important understanding of the behavior of the materials used to optimize the electrochemical performance of the battery. Numerical modeling and data analysis can accelerate this understanding through rapid data generation and in-depth quantitative analysis, however these models are time and computationally consuming.
Over the past decades, significant progress has been made in the field of computer science, and in so doing, artificial intelligence (AI). AI is an abundant source of algorithms for solving problems related to decision-making, computational acceleration, or data segmentation.
Focusing on the calculation of the viscosity of an electrode before drying for different materials, the researchers found a predictive model using functional analysis, applicable to a large number of chemistries commonly used in the battery field (e.g. Nickel Manganese Cobalt Oxide (NMC-111), Lithium-Iron-Phosphate (LFP), and Graphite). This model allows to obtain the result of a simulation 11 times faster than by applying the classical numerical method.
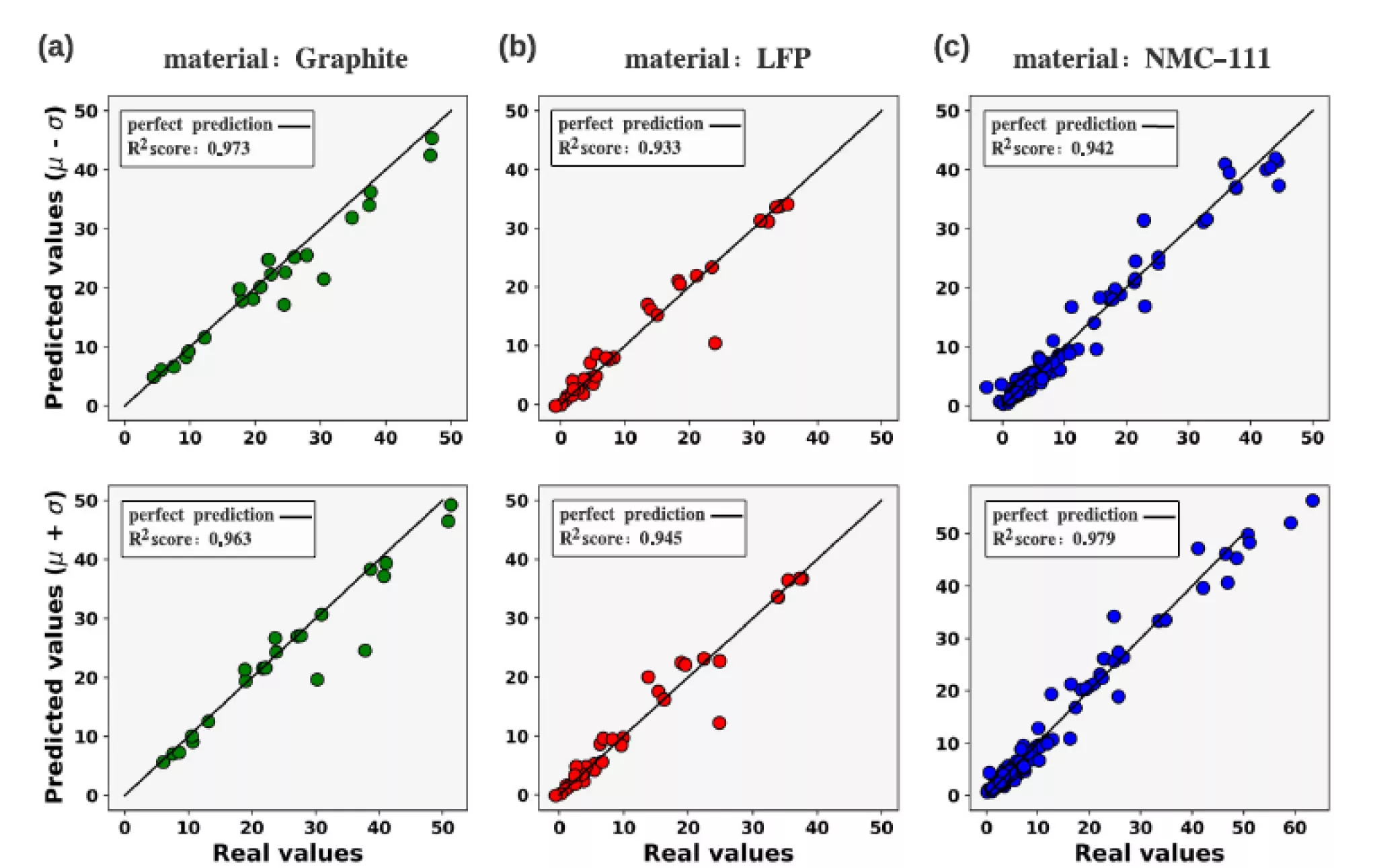
Figure: Regression plots reflecting the accuracy of the model in making predictions independent of the materials used during the simulation.
Thanks to this study, Duquesnoy, et al, were able to predict the result of a simulation from its first numerical values, considerably reducing the time needed for the parameterization of the model. This allowed to characterize the efficiency of the battery during the first steps of its manufacturing, and thus to accelerate the optimization of the battery manufacturing process.
The researchers now hope to be able to apply this type of analysis to any stage in the manufacture of a cell. However, this raises questions about the generalization to all materials, as well as the accumulation of data which is very important.
Read the complete article: https://www.nature.com/articles/s41524-022-00819-2
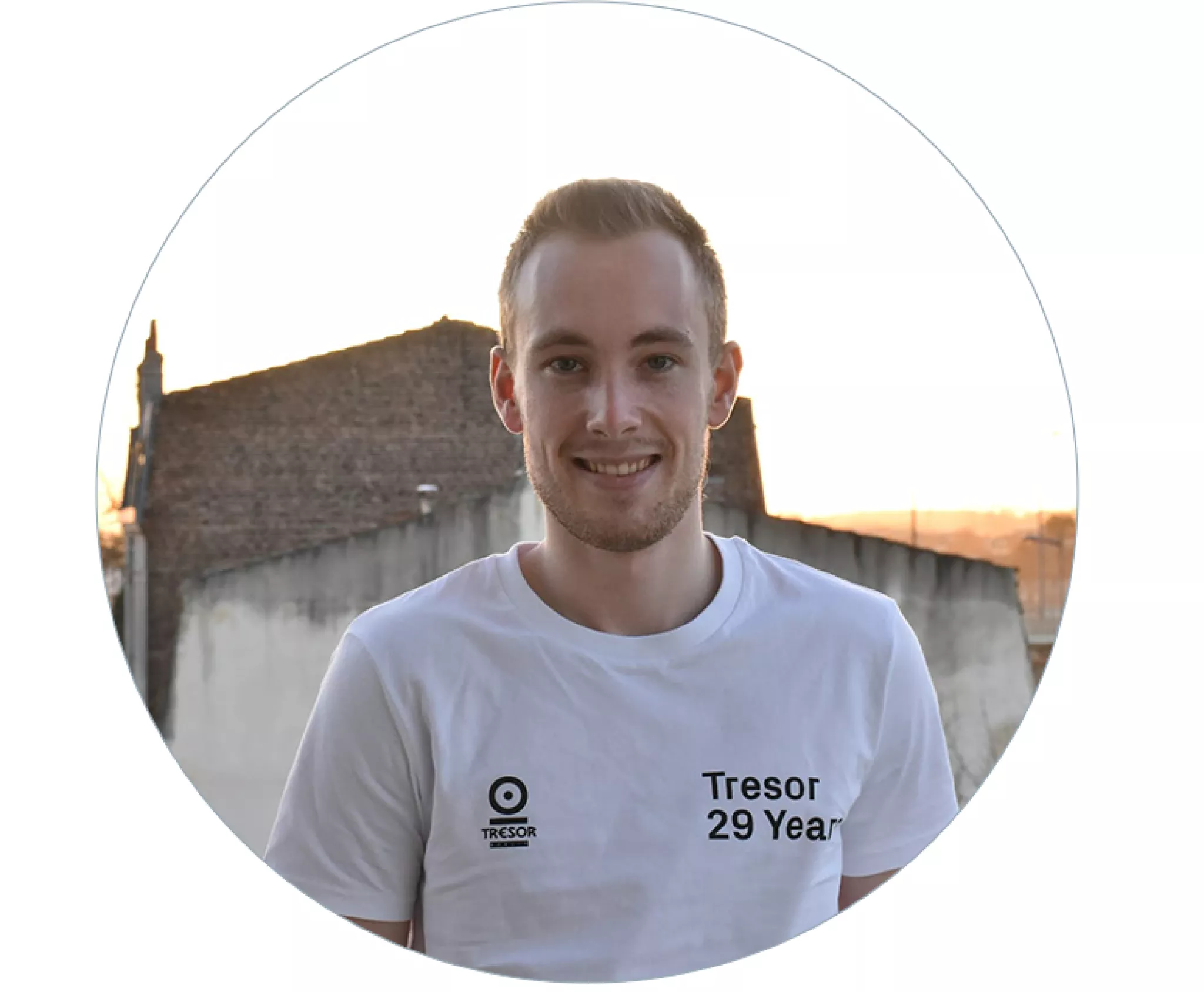
Marc Duquesnoy began his studies with two years of MPSI/MP preparatory classes at the Lycée Chatelet in Douai, in order to obtain a Bachelor's degree in Mathematics at the University of Lille. This allowed him to move on to a Master's degree in Applied Mathematics and Statistics at the same university. His goal was to obtain theoretical and applied skills in mathematics in order to be able to analyze data.
He then joined the Laboratory of Reactivity and Chemistry of Solids (LRCS) as a research engineer to work on the storage of experimental data from battery manufacturing, with an AI component for the analysis of these data. This led him to meet various researchers of different themes, and gave him the opportunity to move towards a PhD with Prof. Alejandro Franco, which was a continuation of his engineering work.
Through his PhD, Marc Duquesnoy seeks to propose data-driven solutions to optimize the manufacturing process of lithium-ion batteries, as well as intelligent solutions for the storage and analysis of data from the modeling of the battery manufacturing process. The objective is to propose a general method of simulation analysis, whatever the materials used.
At the end of these three years, he aspires to get a permanent position as a Data Scientist, if possible in the field of batteries, but he is not closed to other projects as long as they remain in a scientific field.
Thanks to RS2E, Marc Duquesnoy has been able to obtain large project opportunities, meet a whole network of actors, and has so far been able to participate in the publication of several scientific articles.